Gen ai consulting support
Home » Gen ai consulting support7
For Expert Gen ai consulting support
Get in touch with us
Let's break ice
Email Us
1. Auto-Generating Customer Replies
Generative AI understands customer intent. That capability sits at the core of many new customer service use cases for the technology – such as auto-generating customer replies.
Indeed, GenAI applications – like Service GPT by Salesforce – can do this by first understanding the customer query and sieving through various knowledge sources looking for the answer.
Such knowledge sources likely include web links, the knowledge base, CRM, and various other customer databases – which may also allow for personalization.
In trawling these, GenAI automates a relevant customer response, which the agent can evaluate, edit, and forward to customers.
That final part is crucial, keeping a human in the loop to lower the risk of responding with incorrect information and protecting service teams from GenAI hallucinations.
Example: Expanding Agent Replies by Zendesk
Before LLMs burst onto the scene, many people played with generative AI when using tools like Gmail. Indeed, the email tool predicts how a sentence will likely end, and – if it guesses right – the user can hit the “tab” button, and it’ll complete their message.
Embracing the advent of large language models (LLMs), Zendesk built a customer service version of this – on steroids.
Its “expanding agent replies” solution allows agents to type the bare bones of their response and then fleshes it out for them, saving them time in responding to customers across digital channels.
Again, the contact center must plug the solution into various knowledge sources for this to happen – as is the case across many other use cases – and an agent stays in the loop.
Example: Call Note Automation by Sprinklr AI+
Agents often receive a barrage of information, which they must remember. Yet, keeping track of all the critical details is tricky.
As such, many supervisors encourage note-taking. However, even that can impede an agent’s ability to engage in active listening as they multi-task, resulting in increased resolution times.
Sprinklr’s “call note automation” solution aims to overcome this issue by jotting down crucial information as the customer talks.
Agents can refer to these golden nuggets of information when forming their replies – instead of relying on the unstructured, bulky transcript.
Knowing this, they can stay focused on what the customer is saying, not trying to remember what they said previously, which should improve their call handling.
As generative AI monitors customer intent, many vendors have built dashboards that track the primary reasons customers contact the business and categorize them.
With this information, contact centers can understand their primary demand drivers.
This enables the service team to prioritize actions to improve contact center journeys. Such actions may include improving agent support content, solving upstream issues, or adding conversational AI.
Google Cloud’s Generative FAQ for CCAI Insights allows contact centers to upload redacted transcripts to unlock this capability. The tool may also generate conversation highlights, summaries, and a customer satisfaction score to store in the CRM.
Example: Auto-summarization for Genesys Copilot
When a service agent ends a customer interaction, they must complete post-call processing. That typically involves uploading a contact summary and disposition code to the CRM system.
Generative AI solutions can now automate this process, shaving seconds from every contact center conversation and – therefore – saving the service operation significant resources.
CCaaS Magic Quadrant leader Genesys is one vendor to offer such a solution – automating these post-call processes for agents to review, tweak, and publish in the CRM after each conversation.
Moreover, the vendor standardizes the format of each conversation summary. That makes it easier for future agents – handling follow-ups – to get to grips with what happened on the previous call.
Example: The Verint Interaction Transfer Bot
When a contact escalates, the customer must often repeat their problem and the information they shared with the first agent – which is a common source of customer frustration.
Yet, generative AI can help by summarizing the contact so far and sending that to the second support agent or supervisor.
As a result, they can continue the conversation from where it broke down, saving time and preventing the customer from repeating themselves.
The Verint Interaction Transfer Bot does precisely that. No matter if the first agent is a human or a bot, it sends a quick, informative summary rather than an unwieldy transcript.
7. Detecting Customer Service Automation Opportunities
By uncovering customer FAQs, generative AI helps contact centers spot opportunities for conversation automation. Yet, AI Insights by Five9 takes this further.
The solution takes customer conversations and groups them by various traits, like intent.
From there, it applies GenAI and NLP to search for patterns within these groups of contacts, suggesting process and automation improvement opportunities.
In doing so, the tool indicates how often these opportunities present themselves and the possible cost-savings the contact center can make by acting on them.
Example: The Verint Quality Template Bot
After years of call and contact monitoring and CSAT/sentiment analysis, experienced team leaders and quality analysts understand what an excellent customer conversation looks like.
Nevertheless, transferring that knowledge into specific, measurable, and fair quality assurance (QA) scorecard criteria is easier said than done, not to mention time-consuming.
GenAI can help here via solutions like the Verint Quality Template Bot. With this, a QA leader can input simple prompts as to what a top-notch customer-agent interaction looks like on a specific channel.
Then, the GenAI-infused solution will scour historical contacts to spot phrases and behaviors that reflect those prompts on the channel to auto-build a QA scorecard.
Finally, the QA team can review, edit, and finalize that scorecard before repeating the process across other channels (and perhaps specific customer intents).
Example: Manager Assist for Amazon Connect
Many CCaaS providers now offer the capability to automate quality scoring, giving insight into all contact center conversations. This provides a more holistic view of agent performance.
Generative AI takes this further by not only automating “what happened” questions – i.e., did the agent say this or do that? – but additional criteria too.
The Manager Assist for Amazon Connect solution does this by harnessing GenAI to auto-fill more subjective scorecard criteria such as: Did the customer leave the call satisfied? Or: Did the agent offer any concessions?
Alongside this, the solution provides a rationale for the automated answer in case quality analysts, supervisors, or coaches wish to delve deeper or an agent wants to challenge it.
Example: Manager Assist for Amazon Connect
Alongside auto-filling more of the quality scorecard (as above), Manager Assist for Amazon Connect provides an automated agent performance summary for every customer conversation. That summary includes coaching and positive recognition opportunities.
Indeed, in an example that AWS shared, the solution generated feedback such as:
• “The agent could have been more proactive in offering a discount or resolution earlier rather than waiting for the customer to ask. Taking initiative shows commitment to fixing the issue.”
• “The agent did a good job apologizing and taking accountability for the website issues. Expressing empathy for the customer’s frustration is important.”
The innovation also inspires cooperation between quality assurance and coaching teams, who can create a connected learning strategy to bolster agent performance.
Example: NICE Enlighten Actions
Generative AI unlocks several chances to turn insight into action – including insights that conversational intelligence tools uncover.
For instance, NICE uses such tools to detect customer sentiment in real time.
The Forrester Wave CCaaS leader then applies GenAI to monitor the trend in sentiment and alert the supervisor when it drops significantly. They may then swoop in and save the day.
Alongside sentiment, contact centers may harness GenAI to alert supervisors when an agent demonstrates a specific behavior and jot down customer complaints.
Example: Mood Insights by Talkdesk
Many contact center providers offer the capability to score conversations via sentiment.
Some may even share insight on how that sentiment has changed over time so contact centers can decipher – across intents – what is driving positive or negative emotions.
Nifty, but GenAI has allowed vendors to go further. Instead of tagging emotions as positive, negative, or neutral, GenAI-powered sentiment solutions – such as Mood Insights by Talkdesk – capture more specific feelings like frustration, gratitude, and relief.
With this insight, brands can deep dive into how their agents evoke all sorts of emotions and uncover new best practices to coach across the agent population.
Example: Google Contact Center AI Platform (coming soon)
There are many solutions for translating customer chats and messages in real time. Yet, the options for voice have remained particularly limited.
Why? Because they leverage speech-to-text to create a transcript from the customer’s audio. It then passes through a translation engine to pass a written text translation through to the agent desktop.
From there, the agent types out a response, which plays out through a text-to-audio stream.
Technically, this works, and agents and customers can engage in phone conversations while speaking different languages. But, the whole process of having agents type out their replies takes time.
Moreover, those long periods of silence are a real rapport killer.
Enter generative AI, which promises real-time voice translation. OpenAI demonstrated earlier this year how this works using ChatGPT, as shown below.
Unfortunately, there are seemingly no purpose-built solutions for contact centers quite yet. Still, Google has pledged to make such a feature available on its Google Contact Center AI Platform soon. Although, undoubtedly, it will leverage Gemini, not ChatGPT.
Example: Accent Translation by Sanas
Background noise cancellation specialists – such as Sanas and Krisp – generate much of their business in customer service and have long sought ways to bolster their tech stack to increase their presence in contact centers.
GenAI has given them the answer. Take Sanas as an example. It has paired the technology with its audio processing capabilities to turn English spoken with a heavy accent into non-accented American English.
Already, 12 of the top 20 customer service BPOs have leveraged the solution, reportedly cutting agent attrition by up to 50 percent.
Elsewhere, a Japanese telecoms provider is trialing a similar software that modifies the tone of irate customers.
Both use cases have their merit, although ethical concerns have been levied at both, especially regarding accent translation, with critics stating that the tech is “playing into racism”.
Example: CustomerAI by Twilio
To automate customer queries, GenAI-based solutions drink from various knowledge sources. Typically, the contact center knowledge base is the most predominant.
Yet, sometimes, there is no knowledge article for the solution to leverage as the basis of its response.
When this happens, it may flag the knowledge base gap to the contact center management, which can then assess the contact reason and create a new knowledge article.
As a result, the GenAI application has something to work from – as do live agents during voice interactions –enhancing the contact center’s knowledge management strategy.
Example: CustomerAI by Twilio
Alongside spotting gaps in the knowledge base (as above), some GenAI solutions can create new articles to plug them.
CustomerAI by Twilio is an example of such a solution. It understands customer intent, assesses how agents and supervisors have successfully handled such queries, and uses that information to develop a new knowledge article.
A service team may then have a supervisor or experienced agent assess the knowledge article, edit it, and publish it in the knowledge base to keep a human in the loop.
Another advantage of these auto-generated articles is that they’re in the same format, allowing agents to quickly comprehend and action them.
Example: Generative AI App Builder by Google Cloud
Generative AI is changing low-/no-code solutions. It’s allowing users to build applications using natural language alone instead of drag-and-drop tooling.
That will impact many aspects of customer service, and chatbot development offers an excellent early example.
Consider the Generative App Builder embedded into Google’s CCaaS solution: The Contact Center AI Platform. It allows contact centers to build bots in minutes.
Indeed, the developer can explain – in natural language – what information the bot should collect, the tasks it must perform, and the APIs it needs to send data. Then, the platform spits out a bot, which the business can adapt and deploy in its contact center.
Example: Nuance Mix
Now part of Microsoft, Nuance was one of the first vendors to add ChatGPT to its conversational AI platform.
It harnessed the LLM in such a way that if a virtual agent receives a question it hasn’t had training to handle, generative AI provides a fallback response.
The weblinks and contact center knowledge sources that the conversational AI platform integrates with inform the response – helping to automate more customer queries.
Alongside the answer, the GenAI-powered bot cites the sources of information it leveraged, which the customer can access if they wish to dig deeper.
Example: Conversation Booster by Nuance
Automated customer service interactions sometimes break down when customers change their intent halfway through a conversation – confusing the virtual agent.
The Conversation Booster by Nuance uses generative AI to combat this issue as users carry out self-service tasks within the bot. These may include making payments, scheduling appointments, or updating their personal information.
Indeed, the bot detects the intent change and presents a message to refocus the customer, pull the conversation back on track, and improve containment rates.
Example: Conversation Simulation by Cognigy
Like Nuance and Google, Cognigy has pushed the boundaries of generative AI innovation in customer service, as its “Conversation Simulation” tool exemplifies.
The tool bombards virtual agent applications with mock customer conversations to test how well the bot stands up to various inputs.
By pairing this with the Cognigy Playbooks reporting platform, service teams can verify bot flows, validate outputs, and add assertions
The Customers’ Choice conversational AI vendor – as per a 2023 Gartner report – defines an “assertion” as the conditions a bot must meet to pass a test.
Example: Smart Summary Generator by InMoment
LLMs can consume large amounts of information, strip away trends, and convert those into concise, structured takeaways.
InMoment’s Smart Summary Generator does this for customer feedback. Indeed, the GenAI-powered solution first ingests various sources of such feedback – including surveys, conversation transcripts, and online reviews. From there, it generates a trends overview.
If a contact center can continuously feed such a solution with knowledge sources, contact centers can continually monitor customer complaints and act fast to foil emerging issues.
Example: The Expected Net Promoter Score (xNPS) by evaluagent
The Net Promoter Score (NPS) is a common customer experience metric, typically tracked in the contact center.
To calculate a company’s NPS, the customer service team asks the customer: “On a scale of one to ten, how likely are you to recommend our business to a friend or colleague?”
Depending on their answer, the contact center puts the customer into one of three categories: promoters, detractors, and neutrals. It also uses their score to calculate an overall NPS and benchmark performance.
That metric brings significant benefits from segmenting customers to gauging customer loyalty. However, there are drawbacks.
For instance, only a small sample of customers will respond to the NPS survey. Also, customers don’t like filling in surveys; they generally prefer low-effort experiences.
That’s why evaluagent has launched a GenAI-powered solution that analyzes a customer’s contact center conversation before predicting what score they would have left if asked the NPS survey.
While the solution is in beta, the contact center QA provider believes the results are “promising” when tested against real-life NPS data.
Example: Flow Modelling by Cresta
By assessing successful conversation transcripts – across a particular customer intent – generative AI can assimilate the resolution ideal path.
Flow Modelling by Cresta offers such a solution, determining this path based on its impact on various customer experience and business outcomes.
Meanwhile, the capability uncovers the characteristics that lead to successful resolutions. These may inform agent coaching and scorecard creation initiatives.
Example: Industry Benchmarks by NICE
Search engines can auto-generate answers to written questions with generative AI. That functionality may impact several customer service applications.
For instance, consider the knowledge base. When an agent types in a question, it can pop up the answer, so the agent doesn’t have to trawl through articles and documents to find it.
Such a capability may also bring new customer service possibilities to life. The Industry Benchmarks by NICE is an excellent example of this.
At its heart, the solution contains a wealth of anonymized contact center conversation data that NICE has pulled together and used to develop sector-specific benchmarks for many metrics.
Such metrics include customer sentiment, call reasons, automation maturity, and more.
Upfront, the vendor installed a GenAI-infused search engine so service teams can see how they stack up against the competition by simply entering a few written prompts
This article was first published in October 2023, ten months after the launch of ChatGPT, which sent the tech world into a state of frenzy.
In that frenzy, contact center vendors pumped out many GenAI-fuelled features to seize the initial media attention and convince customers that it’s finally time to embrace AI.
Indeed, this list of generative AI use cases for customer service originally included 20 examples.
Yet, upon returning to this article in late August 2024, only five “new” use cases managed to break into the list.
Does that mean that GenAI innovation in the contact center has slowed down? No.
Instead, providers have shifted the focus to feature optimization, not generation. That involves rearchitecting their initial solutions to ensure the best possible performance.
Such optimization initiatives involve allowing the customer to attach their preferred LLM model to power the use case, whether a general LLM – like ChatGPT – or a custom-built model.
Many contact centers will even have multiple LLMs powering numerous use cases across their chosen platform, and – so they know which to use where – some vendors, including Salesforce, will benchmark LLMs against particular use cases.
Alongside that ability to attach a chosen LLM, some providers – like Five9 – allow customers to customize the prompt that powers the GenAI use case. That allows individual businesses to better tailor the generated outputs.
Some vendors have even gone further. Consider NICE. It has built a unique memory graph – EnlightenXM – for each customer. That then feeds a LLM to personalize its outputs.
Expect more innovative thinking to come in 2024/25 and beyond. After all, the low-hanging fruit use cases are already out there. Now, it’s all about creative engineering and differentiation.
~ Testimonials ~
Here’s what our customers have said.
Empowering Businesses with Exceptional Technology Consulting
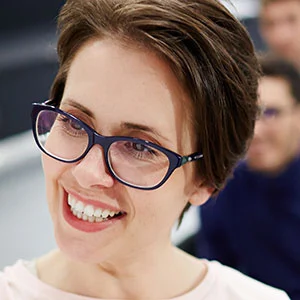
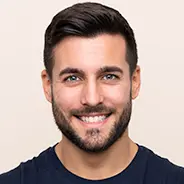
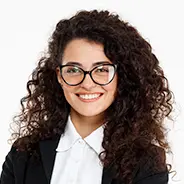